AI for contactless cardiology
Cardiology as a field has seen phenomenal technological advances over the last few decades. A number of tools in cardiology however require sensors and/or electrodes on the human body to capture the cardiac signals and diagnose patients. In this project, we aim to create medical tools that use smartphones and smart speakers to contactlessly detect cardiac conditions.
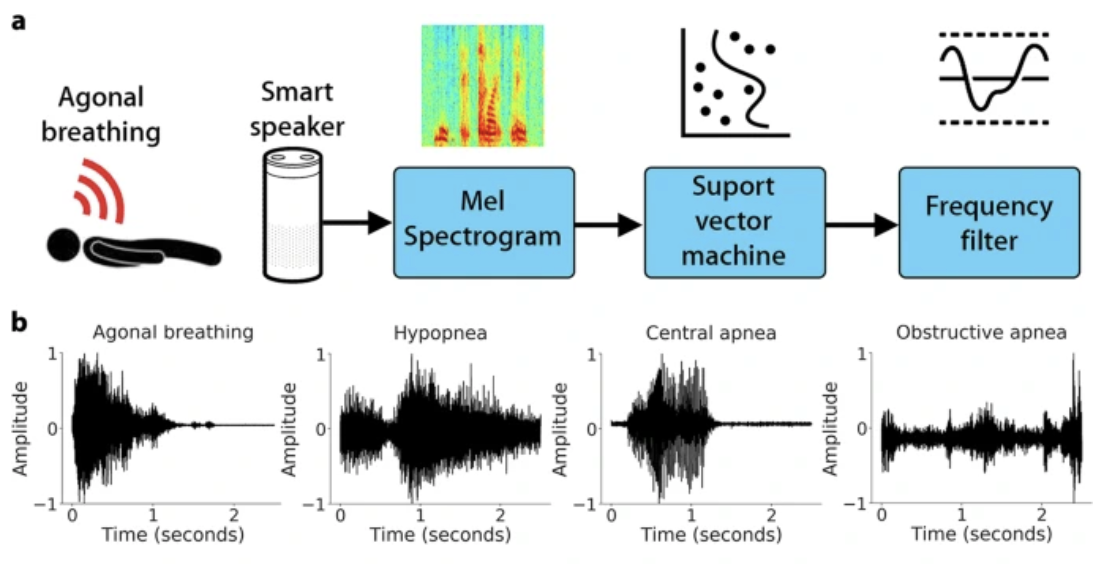
Out-of-hospital cardiac arrest is a leading cause of death worldwide. Rapid diagnosis and initiation of cardiopulmonary resuscitation (CPR) is the cornerstone of therapy for victims of cardiac arrest. Yet a significant fraction of cardiac arrest victims have no chance of survival because they experience an unwitnessed event, often in the privacy of their own homes. An under-appreciated diagnostic element of cardiac arrest is the presence of agonal breathing, an audible biomarker and brainstem reflex that arises in the setting of severe hypoxia. Here, we demonstrate that a support vector machine (SVM) can classify agonal breathing instances in real-time within a bedroom environment. Using real-world labeled 9-1-1 audio of cardiac arrests, we train the SVM to accurately classify agonal breathing instances. We obtain an area under the curve (AUC) of 0.9993 ± 0.0003 and an operating point with an overall sensitivity and specificity of 97.24% (95% CI: 96.86 – 97.61%) and 99.51% (95% CI: 99.35 – 99.67%). We achieve a false positive rate between 0% - 0.14% over 82 hours (117,985 audio segments) of polysomnographic sleep lab data that includes snoring, hypopnea, central and obstructive sleep apnea events. We also evaluate our classifier in home sleep environments: the false positive rate was 0% - 0.22% over 164 hours (236,666 audio segments) of sleep data collected across 35 different bedroom environments. We prototype our proof-of-concept contactless system using commodity smart devices (Amazon Echo and Apple iPhone) and demonstrate its effectiveness in identifying cardiac arrest-associated agonal breathing instances played over the air.
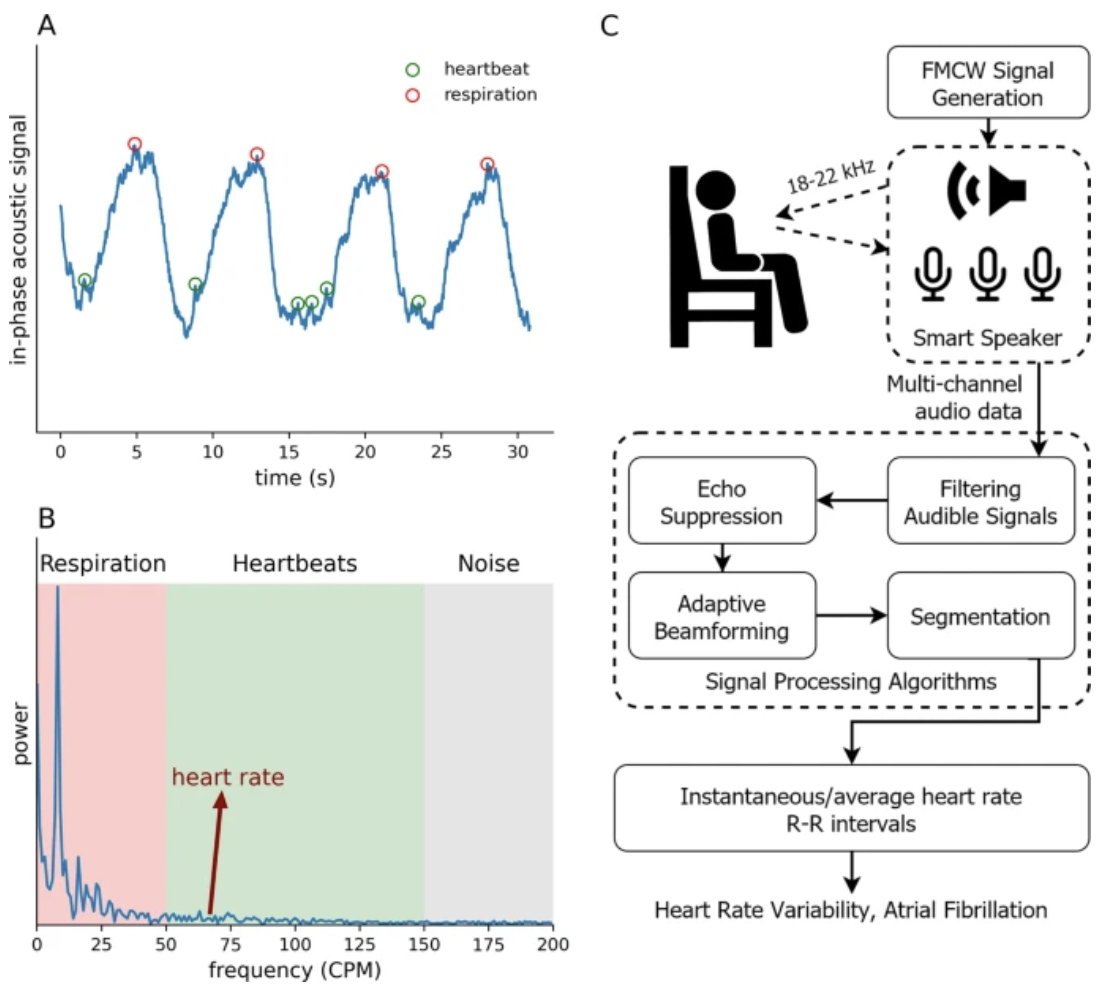
Heart rhythm assessment is indispensable in diagnosis and management of many cardiac conditions and to study heart rate variability in healthy individuals. We present a proof-of-concept system for acquiring individual heart beats using smart speakers in a fully contact-free manner. Our algorithms transform the smart speaker into a short-range active sonar system and measure heart rate and inter-beat intervals (R-R intervals) for both regular and irregular rhythms. The smart speaker emits inaudible 18–22 kHz sound and receives echoes reflected from the human body that encode sub-mm displacements due to heart beats. We conducted a clinical study with both healthy participants and hospitalized cardiac patients with diverse structural and arrhythmic cardiac abnormalities including atrial fibrillation, flutter and congestive heart failure. Compared to electrocardiogram (ECG) data, our system computed R-R intervals for healthy participants with a median error of 28 ms over 12,280 heart beats and a correlation coefficient of 0.929. For hospitalized cardiac patients, the median error was 30 ms over 5639 heart beats with a correlation coefficient of 0.901. The increasing adoption of smart speakers in hospitals and homes may provide a means to realize the potential of our non-contact cardiac rhythm monitoring system for monitoring of contagious or quarantined patients, skin sensitive patients and in telemedicine.
Publications
1. Contactless cardiac arrest detection using smart devices [paper]
Justin Chan, Thomas Rea, Shyam Gollakota, Jacob Sunshine. npj Digital Medicine, 2019
2. Using smart speakers to contactlessly monitor heart rhythms [paper]
Anran Wang, Dan Nguyen, Arun Sridhar, Shyam Gollakota. Communications Biology, 2021